In 2017 I did a postgrad Advanced Statistical Methods course, as it had been 10 years since I had been formally evaluated. As part of my professional development, I like to upgrade my skills in areas that are relevant to the students I work with. As a lot of my work with you pertains to research design, proposals, thesis support as well as research reports, I got a lot out of this course. You can download my journal critique on a Counterfactual Design. It is design to help determine causal inference when using an Observational Method of research outside of the lab. The control group in this design provides 'counterfactual' information; the comparison group where the intervention did not take place can be compared with the observed results with the group which was exposed.
A weakness of this design is that one cannot ever estimate with high accuracy what has occurred in the counterfactual group, as the absence of the intervention in itself, has unpredictable consequences. So, we can never say exactly what might or might not have happened if the intervention were to have taken place.
The paper I review used matched pairs of participants, so that a participant in the intervention group was matched with a participant in the control group, on a bundle of covariates. There is always 'error' or as I prefer to call it 'mystery' (the unknowable) which is what makes life~ Life. The counterfactual design is, I think, an efficient design for data collection and analysis of results, providing one keeps one's feet on the ground when interpreting results. Which should be the case regardless of the design used.
For the particular study of the article critiqued here, I suggested a mixed-method approach~ adding some qualitative elements (e.g., structured interviews) and collecting a broader variety of quantitative covariates (such as level community capacity building, level of spirituality and cultural specific practices).
And I featured the neighbourhood I grew up in for a time, and was again living in whilst writing this paper~ an 'M' area in Cairns where there is high unemployment, youth crime and low SES. I wanted to highlight the 3 M Pride initiative of some of the local youth, as an example of variables that can contribute positively to 'negihbourhood effects'. Or you can read it now, it's posted after the pic~

Neighbourhood Effects Measured with a Counterfactual Design: A Journal Critique Charmayne I. Paul, Advanced Research Methods, Wollongong University, 2017 Observational measurement of the long-term negative outcome causal effects of a childhood spent in a low socio-economic neighbourhood is clouded by selection bias (Harding, 2003; Hedman & van Ham, 2012). Selection bias, the unmeasured factors affecting both neighbourhood of residence and the outcomes for each individual child, are variables that could also explain an association between level of poverty and negative outcomes.
Regression models do not adequately account for unobserved covariates. For example, the FNQ regional city Cairns has 3 ‘M’ areas which are the lowest-poverty urban neighbourhoods in the State outside of Brisbane, with a high rate of high school drop-outs and teen pregnancy; and an average of third generation unemployment; a lack of citizenship role models; high youth crime and inhalant abuse; and poor collaborations across social service providers (Cairns Regional Council, 2017; Karam, Sinclair, & Rackstraw, 2014; Smallbone, Rayment McHugh, & Smith, 2013; Silva, 2014).
In the peer-reviewed study by Harding (2003), the neighbourhood-effects hypothesis was tested using a longitudinal counterfactual model with controlled sensitivity analysis. Although selection bias was not removed or ignored, it was evaluated with regards to explaining how the differing levels of bias emerging from unobserved covariates modified conclusions about the total treatment effect.
Harding’s casual model could explain the high rate of high school drop-out and pregnancy for youth who spent their adolescence residing in high poverty areas the USA from during the 80s-late 90s. This finding was sound in that hypothetical covariates could not explain the associations found between neighbourhood and the two main outcomes. Further, the neighbourhood effects found were larger in magnitude than those in previous literature.
However, a mixed method design would increase robustness of the study as more unobservable and unknown covariates could emerge to explain a causal pathway. Harding’s observational study used a matched (case-control) comparative design which did not randomly assign participants to intervention or control groups. As such ‘identical’ matched pairs of 10-year-olds residing in low or high poverty neighbourhoods were measured on covariates (e.g., annual family income, level of education for parent/s, welfare provisions, and family structure).
Exceptions were made for covariates that the treatment could not affect, for example year of birth, gender and ethnicity. The matching aided comparison of the treatment and control groups and added to the body of knowledge about how those in the treatment group could be matched effectively with untreated participants. The treatment group was defined as those participants aged between 11 and 20 years living in neighbourhoods of poverty.
The main IV was neighbourhood poverty rate during adolescence (See Appendix 1, Results: Flow of participants). Participants living in high poverty areas were defined as being different to those living in low, on observed, unobserved and unobservable outcomes. Matching each treated participant with one or more of the controls enabled the treated participants to be, on average, ‘identical’ to control participants on observable variables prior to the treatment phase. The control participants in this context are the ‘counterfactual’.
The covariates were considered balanced as the participant characteristics able to affect the outcome were well matched. A counterfactual causal framework necessitates deliberation in defining the treatment and the most appropriate covariates for matching participants. Covariate measures did not have reliability and validity values mentioned (CONSORT, n.d.).
The estimand was the true propensity score, which was defined as the unknown probability that a participant would receive the treatment. These scores are not known and are estimated from the observed covariates using a logit or probit model. The score enables the design for analysis of non-randomised observational data that ‘mimics’ a randomised controlled trial (Austin, 2011).
The distribution of observed baseline characteristics in treatment and control groups will be similar, conditional on the balance provided by the propensity score. Previous literature testing matched treatment and control participants on true propensity scores resulted in a balance across observed and unobserved covariates (Austin, 2011; Rosenbaum and Rubin, 1983).
The difference between the two groups following treatment is an unbiased estimator of the average treatment effect (ATE). A limitation of the true propensity score is that the treatment variables must be binary (e.g., low and high poverty neighbourhoods). Binomial probability is the potential for a score to be in one of two states. There is a loss of information in reducing the dependent variable to a nominal level of measurement, which in turn negates statistical power.
Harding also reports that a dichotic DV clouds nonlinear effects, which are not uncommon in the literature on neighbourhood effects. Further, propensity score matching does not control for selection bias with regards to unobserved covariates that do not correlate highly with observed covariates and are different for participants in the treatment group as compared to the control group.
As Harding notes, this biases the treatment effect estimates. However, the causal model is robust for selection bias in non-experimental settings (Rosenbaum, & Rubin, 2003). The teenage pregnancy measurement did not include the time following the birth of the child, so as not to overlap youth who moved to a high poverty neighbourhood after the birth of a child with the neighbourhood effect.
Miscarriages and abortions were not included in the measurement of teen pregnancy, nor mortality of the child which could further inform about potential covariates contributing to tragic outcomes for youth in low-income residencies (c.f., Mason, 2017). Whilst there was no mention in Harding’s study of how sample size was determined, the population of interest (teens residing in high poverty areas in the USA), population of the study (youth living in high poverty in the USA between 1968-1997 whose parents completed the PSID), and the population of causal inference (teens living in high poverty areas of the USA) were clearly delineated.
The Panel Study of Income Dynamics (PSID; 2017) is a longitudinal survey which collects economic and demographic data annually from families (and individuals) in the USA. The survey is ideal to measure neighbourhood effects in that the Geocode Match Files links geographic census data and rates of poverty and other neighbourhood variables. As low-income families are oversampled, the PSID database enables a sample size suitable for analysis of neighbourhood effects.
And, as selective attrition is low, the survey is suited to the longitudinal design and counterfactual model as used by Harding. Respondents born between 1958-1977 that had information pertaining to the outcome variables and who could be matched on census tracts for at least one of the years between the ages of 11 and 20. With regards to the assumptions Harding paid attention to, matching estimators required the same assumptions to be met as for those of regression estimators.
Although the true propensity score matching is a simple method to determine the sensitivity of findings to any unobserved covariates. The causal effect was determined by the difference in outcome between the treatment and “counterfactual” (control) context.
With regards to neighbourhood poverty, exposure to a high-poverty neighbourhood was the treatment. As a single participant was assumed to be unable to experience and not-experience this treatment simultaneously, additional information was drawn on to build a more comprehensive picture. It was assumed that averaging the multiple years of participant data reduced measurement error and better encapsulated the ‘true’ neighbourhood conditions for teens during the treatment period (Harding, 2003; Goldstein, 1968). Thus, the estimates from Harding’s counterfactual model are estimates of the average treatment effect, rather than the effect on each teen per se.
And it was not assumed that all participants receiving the treatment experienced the effect to the same degree (a similar assumption as with regression analysis). Although, it has been suggested that to balance the covariates comparing groups, it is required that an imbalance on unmeasured covariates exist (Brooks & Ohsfeldt, 2013).Sensitivity analysis tested how unobserved covariates that affected the choice of neighbourhood and high school drop-out or teenage pregnancy would change findings about the neighbourhood effect.
The hypothetical covariates suggested by Harding were “parent’s commitment to children” and “wealth”; variables that could affect both the decision of where the family was to live and the probability that the child would drop out of high school or experience a teenage pregnancy. Testing the statistical significance of point estimate changes is inclusive of sampling error, which is ignored when the point estimate of the effect changes due to hypothetical covariates of different strengths being included (Harding, 2003).
Use of confidence intervals of change when unobserved covariates were present was used to report treatment effects. Recommendations to strengthen Harding’s design includes the addition of biopsychosocial variables such as personality traits, self-efficacy, attachment style, social support networks, quality of life of parents/caregivers and teen spirituality (i.e., a belief in ‘something bigger than themselves’), extra-curricular activities of teens, community capacity building, resilience and leadership, as well as the mapping of community resources and access criteria.
Especially, covariates particular to geo-historical area can be informative of a casual model that reflects the true state of being for that high poverty area. For example, inclusion of cultural practices of inter-family adoption amongst Indigenous Australian and South Sea/Pacific Island families in the ‘M’ areas of Cairns in FNQ (c.f., The Adoption Act, 2009). As such, a mixed method design that incorporates qualitative elements to measure lived experiences can reveal emergent factors that contribute to or negate negative neighbourhood effects (see Appendix 2).
The inclusion of qualitative data into the design could counter the weakness of the counterfactual model in being a complex matching process, as emergent factors could provide better matched covariates for a greater number of treatment cases. Given that Harding concludes that neighbourhood effects are related to long-term developmental processes, greater inclusion of contextual variables could better inform neighbourhood effects on negative teen outcomes that do occur.
As well as identifying factors of neighbourhood effects that result in positive teen outcomes. Ultimately it is vital to learn more about the causal mechanisms affecting negative and positive teen outcomes due to neighbourhood effects, and to more accurately measure these mechanisms, to inform social science research design, subsequent policy decision making, and to increase youth awareness of their potential for creating change in their lives.
References Austin, P.C. (2011). An introduction to propensity score methods for reducing the effects of confounding in observational studies. Multivariate Behavioural Research, 46(3), 399- 424.https://www.ncbi.nlm.nih.gov/pmc/articles/PMC3144483/
Brooks, J. M. & Oat, R. L. (2013). Squeezing the balloon: Propensity scores and unmeasured covariate balance. Health Service Research, 48(4), 1487–507. https://www.ncbi.nlm.nih.gov/pubmed/23216471 Cairns Regional Council. (2017). Community Development Strategic Plan, 2017. Retrieved June 3rd, 2017 from, http://www.cairns.qld.gov.au/community-environment/community-services/comdev-strategic-plan
CONSORT. (n.d.). Consort 2010: a Outcomes. Retrieved June 3rd, 2017, from http://www.consort-statement.org/checklists/view/60020-n-of-1-trial/1154-outcomes
Goldstein, H. (1968). Longitudinal studies and the measurement of change. The Statistician, 18(2), 93-117.
Harding, D. J. (2003). Counterfactual models of neighborhood effects: The effect of neighborhood poverty on dropping out and teenage pregnancy. AJS, 109, 3 676–719.
Hedman, L. & van Ham, M. (2012). Understanding neighbourhood effects: Selection bias and residential mobility. Retrieved June 3rd, 2017 from https://link.springer.com/chapter/10.1007/978-94-007-2309-2_4#page-1
Karman, J., Sinclair, G. & Rackstraw, L. (2014). Dignity, diversion, home and Hope: A review of interventions for volatile substance misuse in regional North Queensland. Retrieved June 3rd, 2017, from https://www.dpmc.gov.au/resource-centre/indigenous-affairs/dignity-diversion-home-and-hope-review-interventions-volatile-substance-misuse-regional-north-queensland
Mason, G. (2017). Residents recall horror… Retrieved June 3rd, 2017 from http://www.cairnspost.com.au/news/cairns/residents-recall-horror-of-discovering- unnamed-babys-torso/news-story/34e596d7289cf687c88ea27d179b3351
The Panel Study of Income Dynamics (PSID). (2017). Retrieved June 3rd, 2017 from https://psidonline.isr.umich.edu/
Rosenbaum, P. R., Rubin, D. B. (1983). The central role of the propensity score in observational studies for causal effects. Biometrika. 70(1): 41–55. doi:10.1093/biomet/70.1.41
Silva, K. (2014). Cairns mother Raina Thaiday … Retrieved June 3rd, 2017 fromhttp://www.abc.net.au/news/2017-05-04/mother-killing-eight-children-in-cairns-wont-face-criminal-trial/8489830
Smallbone, S., Rayment McHugh, S., & Smith, D. (2013). Preventing youth sexual violence and abuse in West Cairns and Aurukun. Retrieved June 3rd, 2017, from https://www.datsip.qld.gov.au/resources/datsima/programs/ysv-report.pdf
Appendix 1
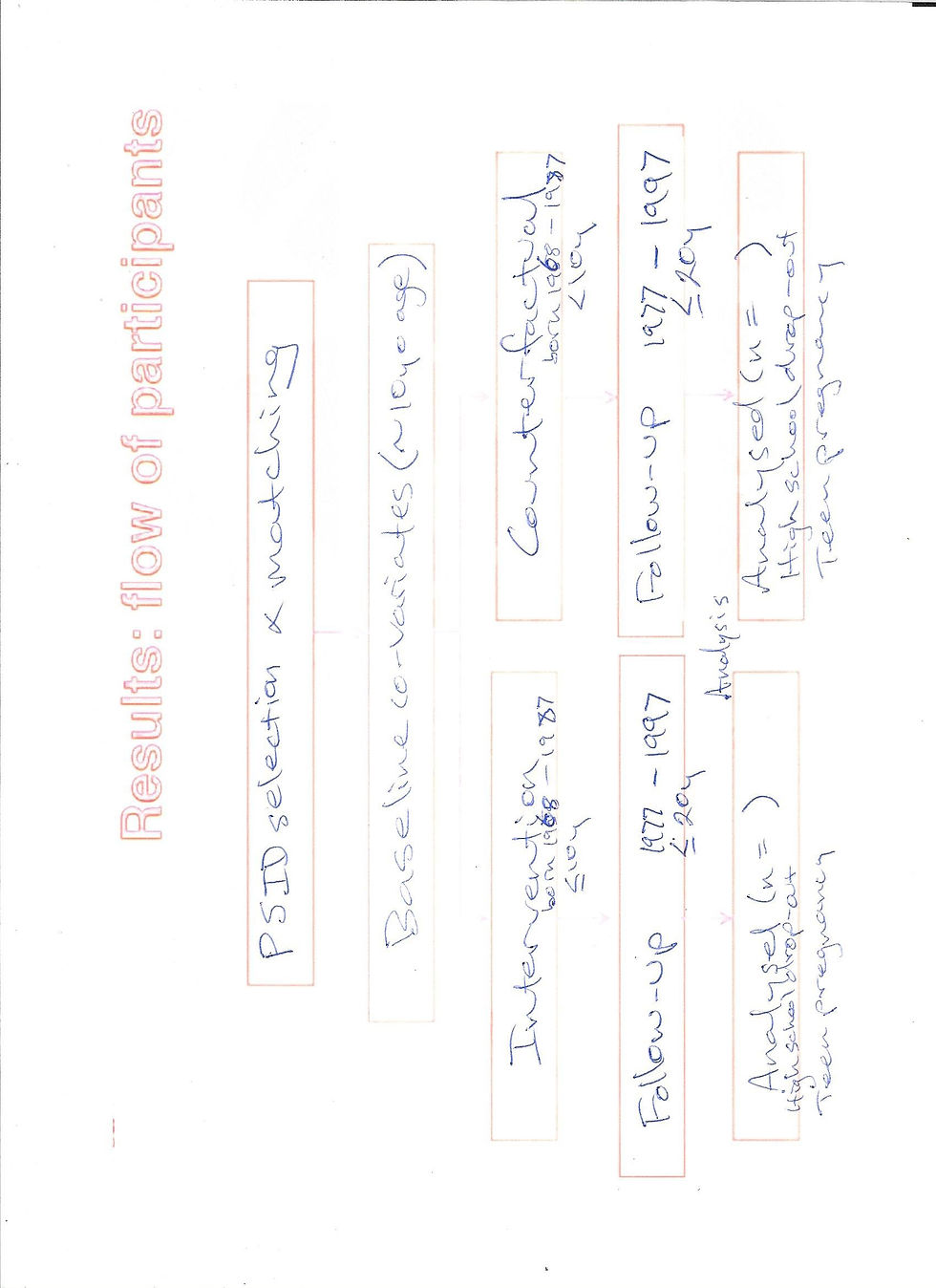
Figure 1. Harding’s participant flow aligning with the CONSORT 2010 template; n for each group is unknown due to the cryptic censure tract choice procedure.
Appendix 2
I reside (and grew up in) in an ‘M’ area of Cairns, FNQ. Earlier this year within the Cairns West catchment, a group of youth mentored by the community PCYC dropped a hip-hop tune and music video titled, 3M Pride.
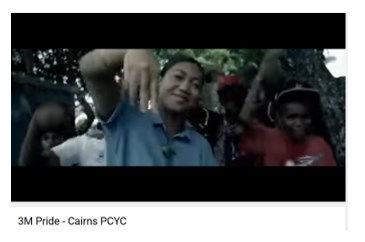
Inspired by the sense of ownership and belongingness of the youth, I immediately shared their project on my Facebook pages, @Reuse and Recycle, Cairns and @Psych n Stats Tutor. And I printed myself a sign for my fence that featured the phrase ‘3 M Pride’ to encourage residents to participate in the youth directed culture of taking pride in the neighbourhood. I placed the sign above my ‘food is free’ plant giveaways to demonstrate a way to put ‘pride into action’.

Three months after putting up my sign, 3M Pride stickers were put in my letterbox.
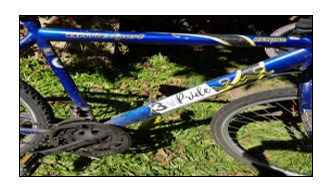